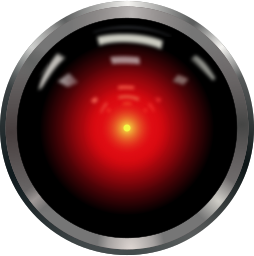
Artificial Intelligence
The AIDA research group has been involved for over twenty years of the Artificial Intelligence field. The specific focus on Machine Learning and Pattern Recognition has developed both in the speculative theorist approach, gaining vertical skills on model optimization, both through application to real problems, especially in the Healthcare field. In particular, attention is paid to decision support systems concerning the diagnosis of neurodegenerative diseases through the analysis of handwriting and the diagnosis in mammography and ophthalmology by way of the analysis of images with a deep learning approach.
Movement Analysis for Health and Biometric
Movement is being investigated in biomechanics, neuroscience, psychology, and artificial intelligence. The availability of low cost and pervasive devices for recording movements (wearable devices, smartphones, tablets, cameras, etc.), together with machine learning methods for the quantitative and automatic analysis of movement, has put forward the development of systems for user authentication, medical diagnosis, and rehabilitation monitoring. The research group models and analyses the movement contributing to both the understanding of human movement and the development of new applications.
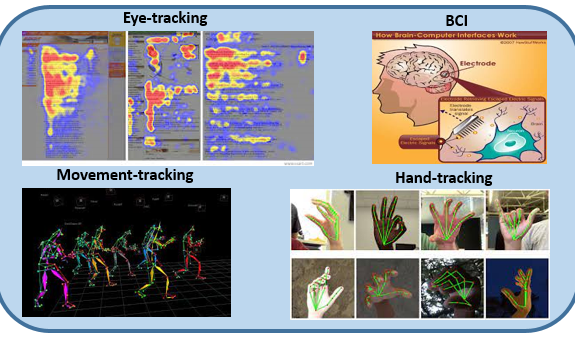
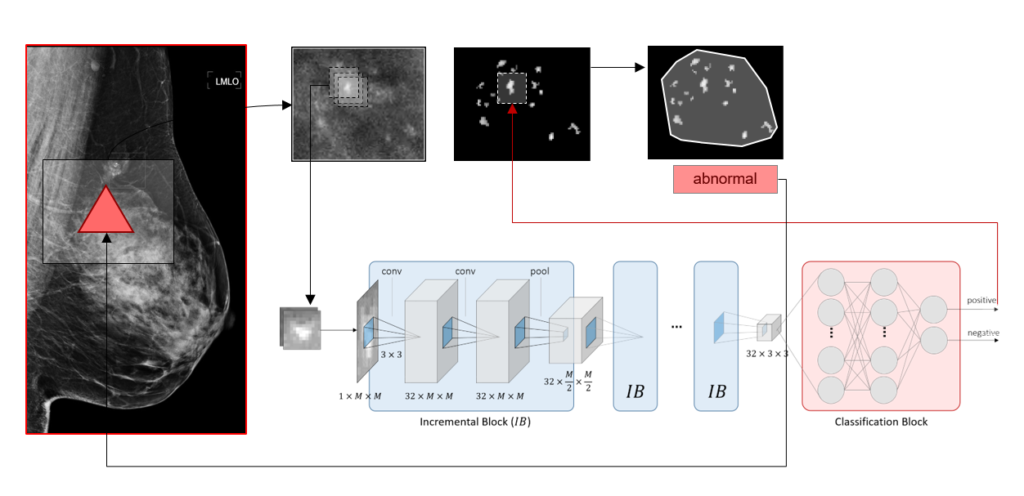
Medical Image Analysis
This research focuses on designing and implementing Computer Aided Detection and Diagnosis (CAD) systems for medical images of various modalities, including mammograms for breast cancer prevention and diagnosis and retinal images for diabetic retinopathy detection. To this end, both machine and advanced deep learning techniques are employed, such as deep cascades of boosting classifiers and deep convolutional and attentional neural networks. Particular emphasis is placed on the detection of small lesions (such as microcalcifications in mammograms and microaneurysms in retinal images) which is characterizable as a severely imbalanced classification and detection problem, and on lesions that appear at different scales (such as masses in mammograms and exudates in retinal images). On top of this, medical images denoising and noise-stabilization techniques are also investigated and tailored for the specific image modality.
Brain Computer Interfaces
This research focuses on using low-cost noninvasive Brain Computer Interfaces (BCIs) for acquiring EEG signals to be analyzed with ad hoc designed deep learning techniques to implement various applications including motor imagery for external devices control (wheelchair, robotic arms, domestic robots, etc.) and assisted neuromotor rehabilitation. EEG signals acquired with noninvasive low-cost BCIs pose several challenges for deep learning including low signal-to-noise ratio, extreme inter-subject and inter-temporal variations, data scarcity, to name a few. All these issues are targeted in the design of custom deep neural networks architectures such as deep learnable versions of Filter Bank of Common Spatial Patterns (FBCSP) and deep learnable bandpass filters combined with existing lightweight architectures for EEG signals (such as EEGnet).
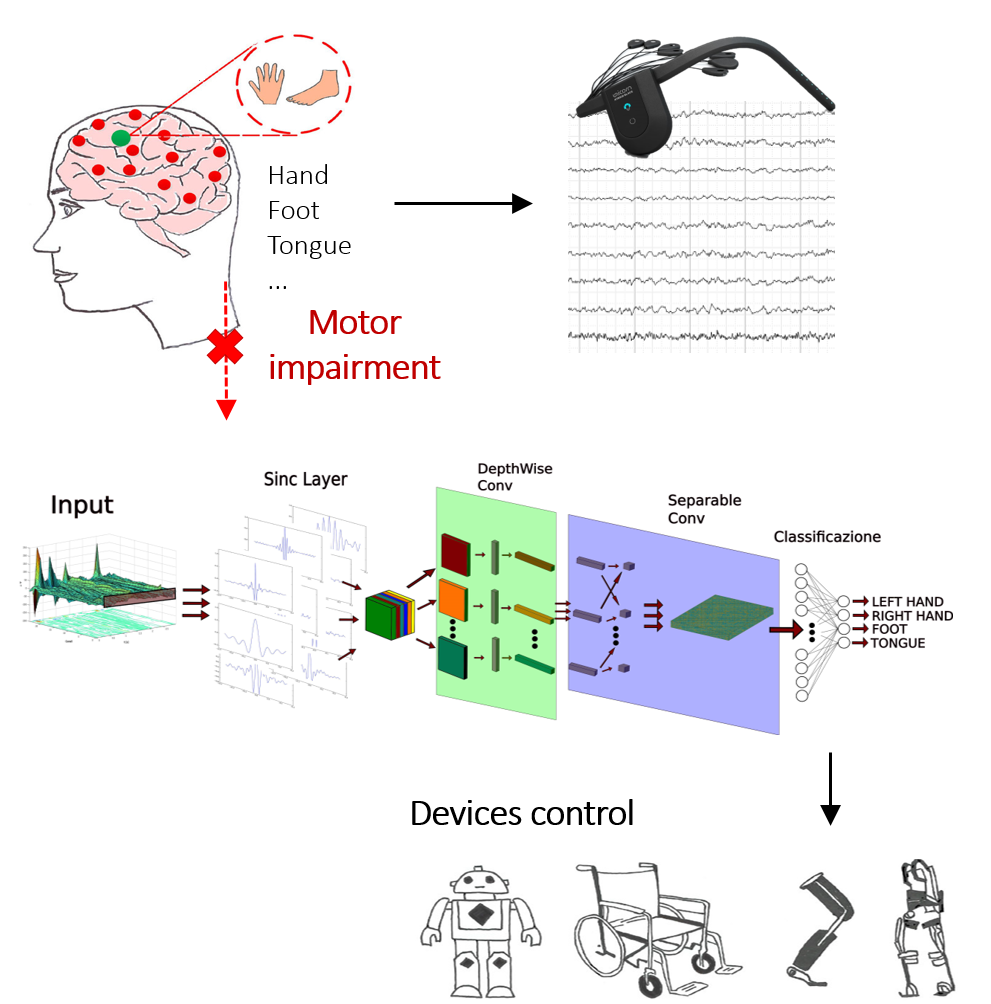
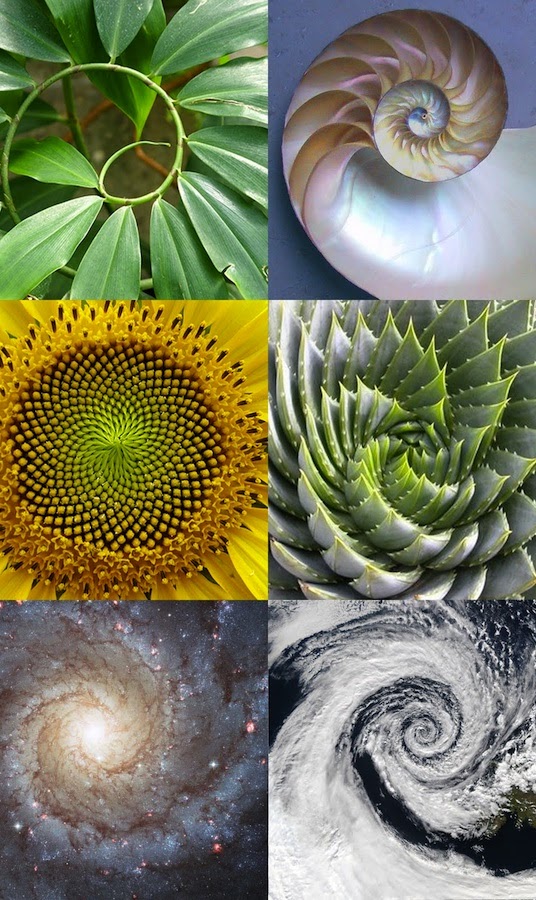
Mathematical Modelling & Pattern Formation (Deborah Lacitignola)
The development and analysis of mathematical models in the socio-epidemiological field, especially related to the Covid-19 epidemic, is a very hot topic. As a part of the Mathematical Physics sector (MAT/07) this issue is actively carried on by the means of nonlinear dynamical systems and bifurcation theory. Along with the qualitative characterization of different dynamical scenarios, particular attention is given to the control and management of complex phenomenologies as hysteresis and chaos. In addition, a gallery of pattern formation problems with a wide range of applications (from electrochemistry to biology) are studied within the framework of the reaction-diffusion systems on fixed and evolving domains. The occurrence of diffusive instability, Hopf instability and their interplay is investigated and related to the emergence of self-organised patterns and complex spatio-temporal dynamics.
“Learning” sensors
A smart sensor is a device equipped with one or more analog sensors that measure the quantities of interest, equipped with analog electronics for signal conditioning and its conversion into digital, and finally equipped with a communication interface.
At the University of Cassino, in collaboration with the company Sensichips S.r.l. and the University of Pisa, the development of a modern sensing and data processing platform called SENSIPLUS Deep Machine (SDM) is underway, which represents a complete system for the detection and recognition of volatile substances or substances dissolved in water.
The SDM is presented as an IoT-ready device suitable for both Smart Cities and Industrial areas and is equipped with an engine for classification/regression created with Machine Learning techniques. From an architectural point of view, SDM can be described as a two-layer architecture: one hardware and one software. The hardware layer is centered on the SENSIPLUS chip and from a commercial microcontroller; the software layer was developed in our laboratories and is based on the most modern machine learning frameworks, allowing the use of different algorithmic solutions: from the simplest and lightest ANN, up to the heaviest and most complex CNN or LSTM. This variety of solutions allows you to choose the right trade-off between accuracy and computational burden.
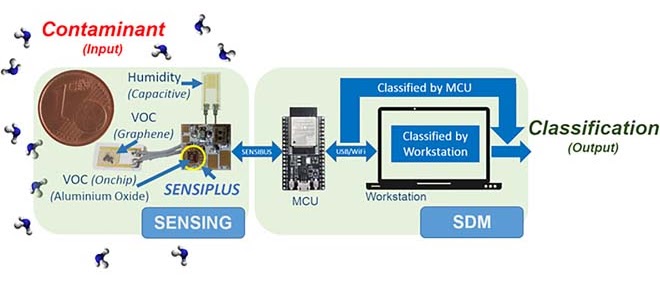
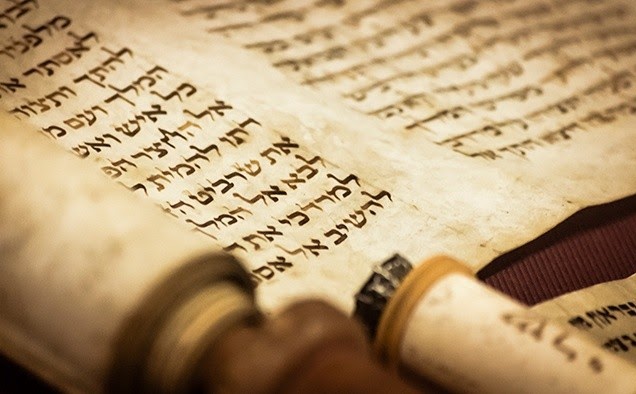
Artificial Intelligence for Cultural Heritage
Cultural Heritage (CH) needs to be preserved and promoted, it is a sector that has to deal with a vast amount of complex and different data, where diagnostics and preservation are fundamental to determine the state of conservation of historical monuments. Not an easy task for humans at all, that’s why Artificial Intelligence (AI) offers solutions in order to objectively and efficiently manage and analyse the amount of information.
The trend to digitize CH increased a lot over the last few decades and it led researchers towards the development of automated tools to study cultural heritage and assist the experts in the field.
The AIDA research group studied AI and Pattern Recognition techniques to support paleographers in the study and analysis of ancient documents. Indeed it has been involved in digital paleography aimed to writers’ identification in medieval manuscripts and on greek papyri.
Battery
At the Artificial Intelligence and Data Analysis Lab, our research on lithium batteries focuses on enhancing their performance and reliability through advanced data-driven techniques. We specialize in estimating the State of Charge (SoC) and State of Health (SoH) of lithium batteries, ensuring accurate assessments of battery capacity and longevity. Our work includes developing parametric models that capture the dynamic behavior of batteries under various operating conditions. Additionally, we conduct in-depth studies of battery chemistry to understand and optimize the electrochemical processes involved. Our team also focuses on fault detection, identifying potential issues early to prevent failures and extend battery life. By leveraging artificial intelligence and data analysis, we aim to contribute to the advancement of lithium battery technology, promoting efficiency and sustainability in energy storage solutions.
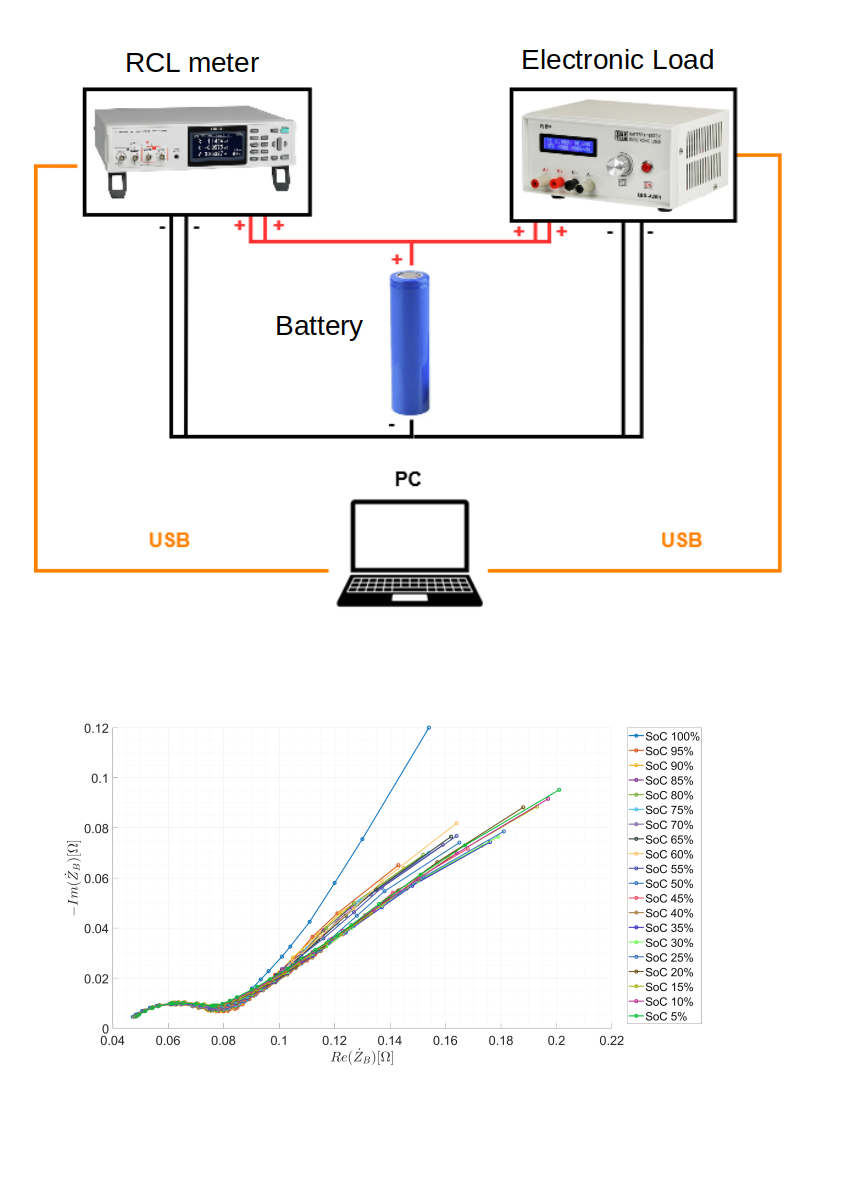